Luc Tuymans through the lens of AI
Luc Steels.
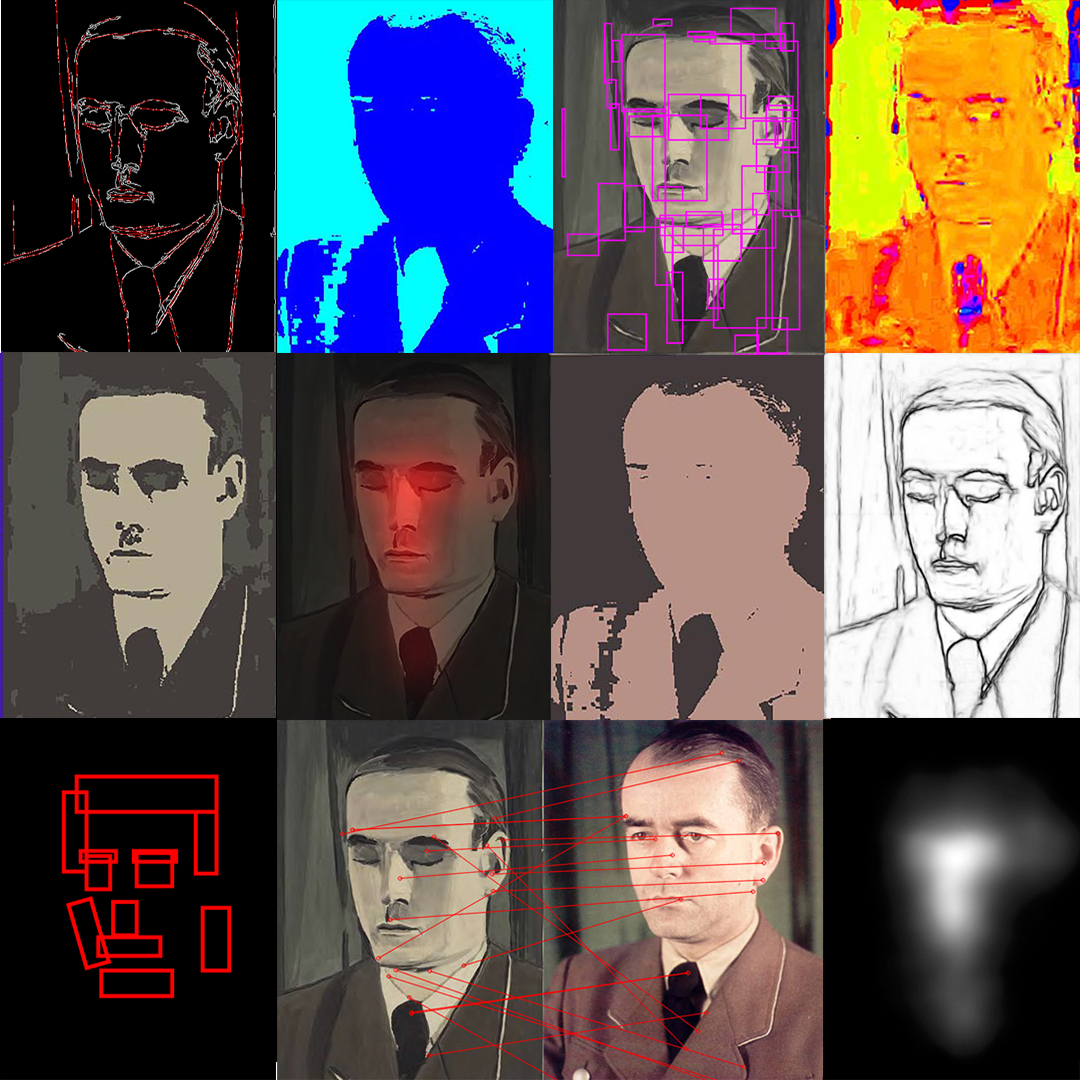
AI (Artificial Intelligence) researchers try to understand the structures and processes that underlie intelligence and use that insight to build practical applications. Much has already been achieved. But much remains to be discovered. The way to make these discoveries is to explore domains where human intelligence reaches its most exceptional manifestations. The creation and interpretation of artworks is certainly one of them.
Recently I had the opportunity, facilitated by the Brussels cultural institution BOZAR and the Gluon Foundation, to collaborate with the renowned contemporary Flemish painter Luc Tuymans as part of the STARTS program of the EU that promotes interaction between art and science. I took this opportunity to advance the MUHAI research agenda and help my AI colleagues open their eyes to the arts. But I also wanted to give people from the arts and the wider public insight into what AI stands for and what it can and cannot do. This is necessary because, at least in my view, AI is now overhyped, with unrealistic expectations and misunderstanding of the complexity and enormous challenges that we still face to build artificial systems that approach human intelligence.
Other scientists investigating art, such as chemists or physicists, put a work of art on their dissection table and use the measuring instruments of their field to understand the material aspects of a work and thus solve puzzles about provenance and painting technique. In contrast, AI is more comparable to psychology. It is interested in the cognitive processes that take place when viewing, experiencing and interpreting a work of art on the one hand and the conception and creation of a work of art on the other. Psychologists do this through observations and empirical experiments. AI researchers make models.
AI models do not simulate exactly how a person does it. They are functional models, models that yield the same result as a specific human cognitive capacity but often in a very different way. For example, they may focus on recognizing that a painting represents a face, or that this face is depicted as being angular, that the color palette used in a painting emphasizes greyish colors, or that the person depicted in the painting is holding his lips tightly together. AI is artificial intelligence the way an airplane is an artificial flying machine. An airplane can really fly in the physical world and lift weight but it doesn't do it by flapping its wings like a bird. The validity of a psychological theory is determined by how well it covers the collected data and makes predictions about new data. The validity of a functional AI model is tested by making a computer implementation, possibly embedded in a robotic body, which demonstrably achieves the function being examined.
Many people entertain the idea that AI is a monolithic physical force, like electricity or gravity, but that is not the case. AI is about algorithms that must work together to realize even basic cognitive functions. We already know thousands of these algorithms and many more remain to be discovered. An AI algorithm usually needs a knowledge base that it consults to achieve its function. For example, if an algorithm wants to recognize faces, it must of course first know the distinctive features of each face. These knowledge bases have to be created by an AI algorithm as well (this is what we call 'learning' in AI parlance) because it is often impossible to collect, disassemble and formalize all the necessary knowledge by hand. For example, an algorithm learning to recognize the parts of a face will first be presented with millions of faces, with annotations where the parts of each face are located. Based on these data, the algorithm then gets to work creating a knowledge base that can then be used to process new faces.
Many AI algorithms used today in the context of art are about the form of a work and its style. For example, it is popular to show all the paintings of a particular artist, such as Claude Monet or Vincent van Gogh, to a learning algorithm, so that it can build a statistical style model and to then create a new work in the style of the artist. The results achieved using this approach are astonishing because the results are very similar to an original work by the same artist, particularly when neural network techniques known as Deep Learning algorithms are used. But note that these experiments do not go into the meaning of a work, for example why a particular figure is depicted, why certain colors have been chosen or why the face has been strongly distorted. Deep learning and other machine learning algorithms have no idea what the painting represents. The ability to cope with meaning is currently the glass ceiling of AI, the barrier that distinguishes human from machine intelligence.
And it is precisely the goal of the MUHAI project to break through this barrier.
In this spirit I tried out a completely different approach on art and AI, focusing on the question how a work of art evokes and expresses meanings. This meant that I had to go in two steps: First find AI algorithms that can discover visual features, for example that a face is depicted as being more angular than the original. And then look for AI algorithms that can figure out the meaning of these features, for example by calling on the immense semantic networks that have been developed in symbolic AI in order to develop search engines or language applications.
Early on I decided to focus on the solo exhibition 'La Pelle' by Luc Tuymans in the Palazzo Grassi in Venice, which was on view from the summer of 2019 to the winter of 2020. Together with several collaborators (in particular Bjorn Wahle and Sinem Aslan) I started to apply all kinds of AI algorithms to the entire collection of the 80 works in the exhibition. Via Studio Luc Tuymans I had access to the original source material and I was able to have a number of conversations with Luc Tuymans himself. These conversations, as well as Tuymans' clear writings, shed a clearer light on his artistic method. An important element of that method is that Tuymans almost always works with existing source material. So it is relevant, and very enlightening, to compare a painting with its source. In this way the transformations that the artist has made come to the fore and that in turn helps us to investigate why the artist chose these specific transformations, in other words which transformations are signifiers, i.e. carriers of meaning.
After some thorough preliminary studies, I concentrated on one work, Secrets, and built an exhibition about it that is on view at BOZAR from 3 April to 3 May 2021. The exhibition shows the two stages in the interpretation of a work of art: the visual and the conceptual. The visual processing starts from the image and tries to find areas of focus that the human eye naturally tends to, as well as edges, lines, shapes, geometric figures, colored areas, contrasting zones, and more (see Figure 1). Such an analysis yields points and areas in the image that pattern recognition algorithms can then try to relate to known objects, such as parts of a face, or to their features, such as that the eyebrows are thick or the nose sharp.
Figure 1. Some examples of image processing applied to the work Secrets. From left to right: (i) The painting itself, (ii) the focal region according to the MSI algorithm, (iii) the focal region according to the Spectral-residue algorithm, (iv) lines found by the TIN algorithm, (v) comparison of the lines between the painting and the original image.
The conceptual processing next tries to derive factual information and emotional associations from what is depicted and consults knowledge graphs, dictionaries, thesauri, ontologies and other semantic resources on the internet to put the painting into its context: the historical context, the cultural symbolism and the broader context of the exhibition 'La Pelle 'in the Palazzo Grassi. The conceptual processing also uses as input the title of the work (Secrets) and the text of the catalog, which contains keys for searching the web. As the interpretation process unfolds, all these AI algorithms together build a very large 'narrative network' that acts as a coherent description of the painting from multiple points of view. This network opens our eyes to the richness and semantic complexity of the painting (Figure 2).
Figure 2. Fragment of the narrative network built for Secrets. The network contains associations based on image processing but also historical references, biographical data, references to the exhibition as a whole, etc.
Has this interaction between art and science been successful? I think so. Most scientists are not used to experiencing art in a profound way and are amazed by the multitude of meanings and layers of processing that dedicated viewers recognize. But also for people from the art sector, including Luc Tuymans himself, this experiment is revealing because it illustrates the nature and complexity of AI and what associations and insights machines can already gather today about an artwork. At the same time, this experiment illustrates that the current state of AI is miles away from how humans perceive and interpret art. Claims, such as by Ray Kurzweil, that AI is going to outdo humans in the coming decades sound ludicrous when you examine the tremendous richness of the human mind as manifested in the arts through the 'cognitive microscope' offered by AI algorithms.
References
Steels, L. and B. Wahle (2020) Perceiving the Focal Point of a Painting with AI: Case Studies on works of Luc Tuymans. Proceedings of the 12th International Conference on Agents and Artificial Intelligence - Volume 2: ICAART, 895-901, Scite Press, Setubal Portugal.
Sinem, A. and L. Steels (2021) Identifying centres of interest in paintings using alignment and edge detection. Case studies on works by Luc Tuymans. In: International Workshop on Fine Art Pattern Extraction and Recognition (FAPER 2020). Proceedings of the International Conference on Pattern Recognition (ICPR) Part III. LNCS 12663. Springer Verlag, Berlin.
[A Dutch version of this note is published in: Monard, E. (ed) (2021) Kunst en wetenschap in symbiose. Viewpoints of the Royal Flemish Academy for Sciences and Arts of Belgium, Brussels.]
More Articles
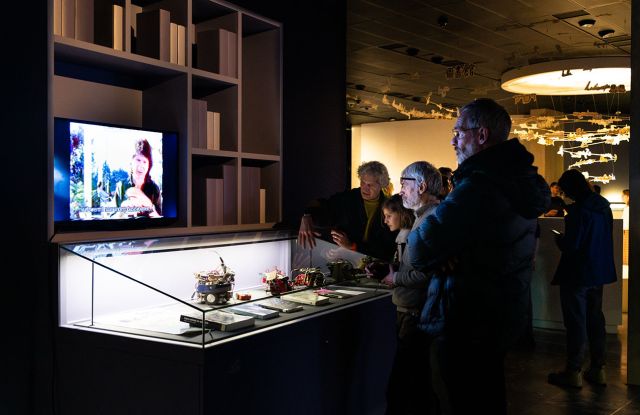
Science on the edge of chaos
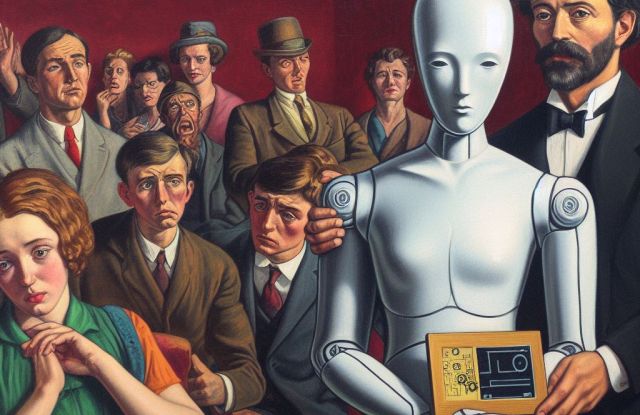
AI, the winning artist?
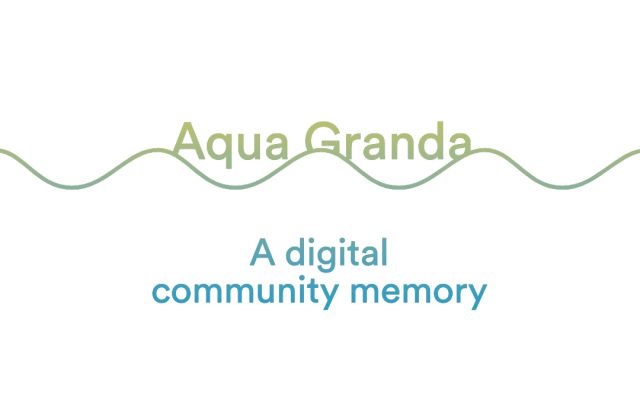